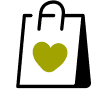
Matchify
Your AI Shopping Assistant
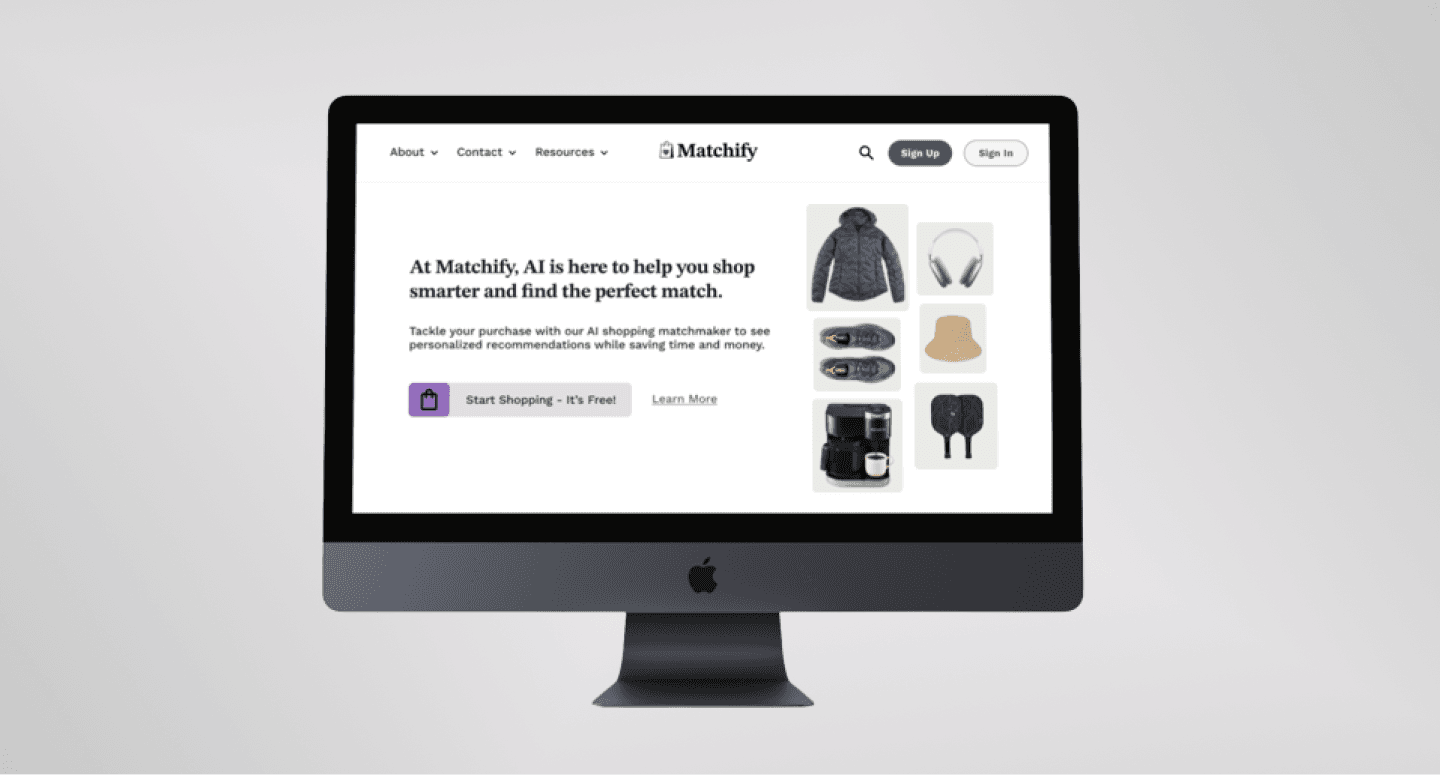
Work
Matchify
Designed a web platform for
better shopping experience using generative AI.
Matchify is a web platform designed to use generative AI to help make the process of online shopping easier and less overwhelming. Think less tabs open and fewer abandoned shopping carts, boosts buyer confidence when making online purchases, particularly in categories with a large amount of product offerings.
Info
Role
UX Researcher
UX Designer
Team
Aditya Etiyala (Me)
Jenna Lee
Grace Maloney
Ethan Baccam
Melika Ziba
Duration
4 months
Mentor
Debra Kumar
Problem
What needs fixing?
How might we help consumers be more satisfied when making online purchases, particularly when shopping for an item in a category with a large amount of product offerings?
Outcome
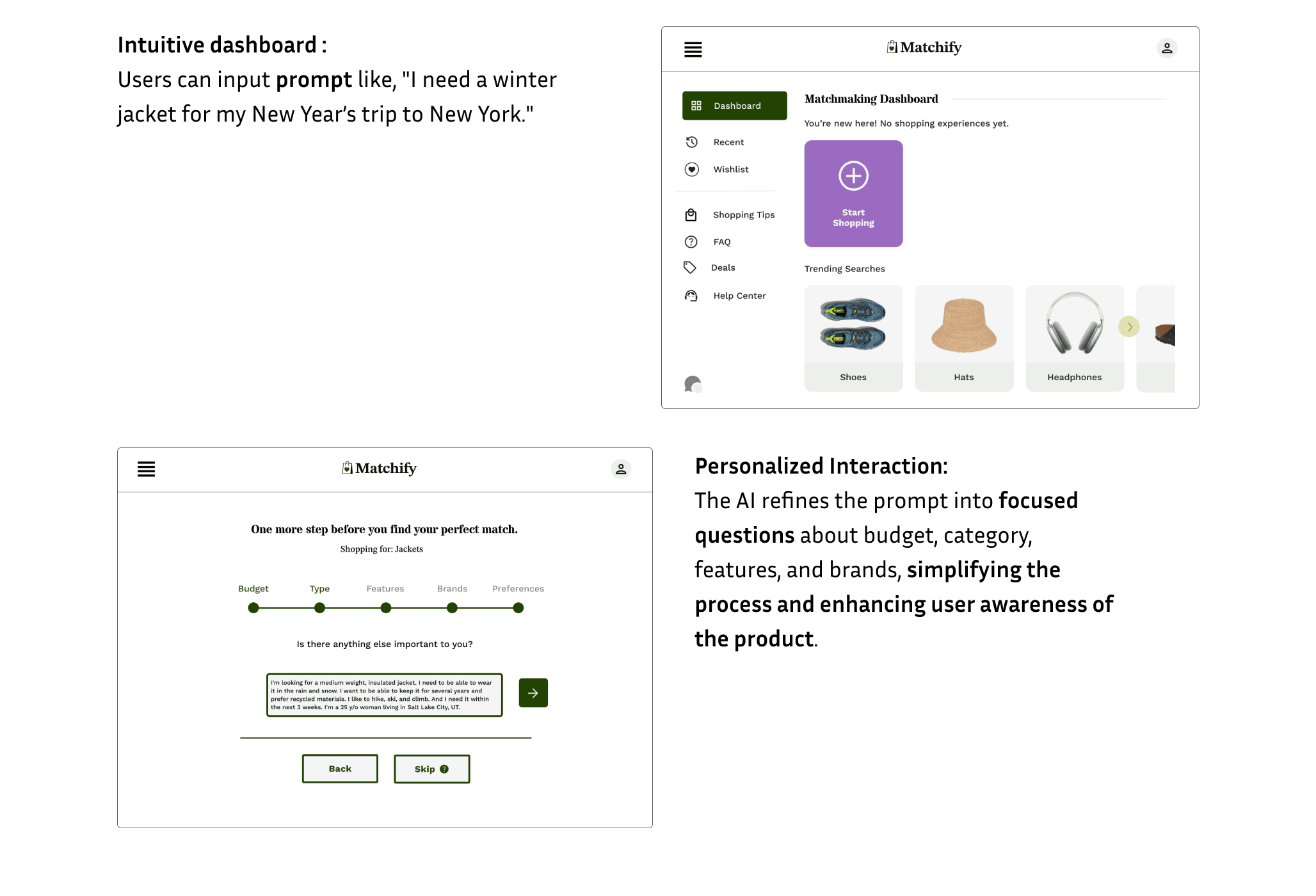
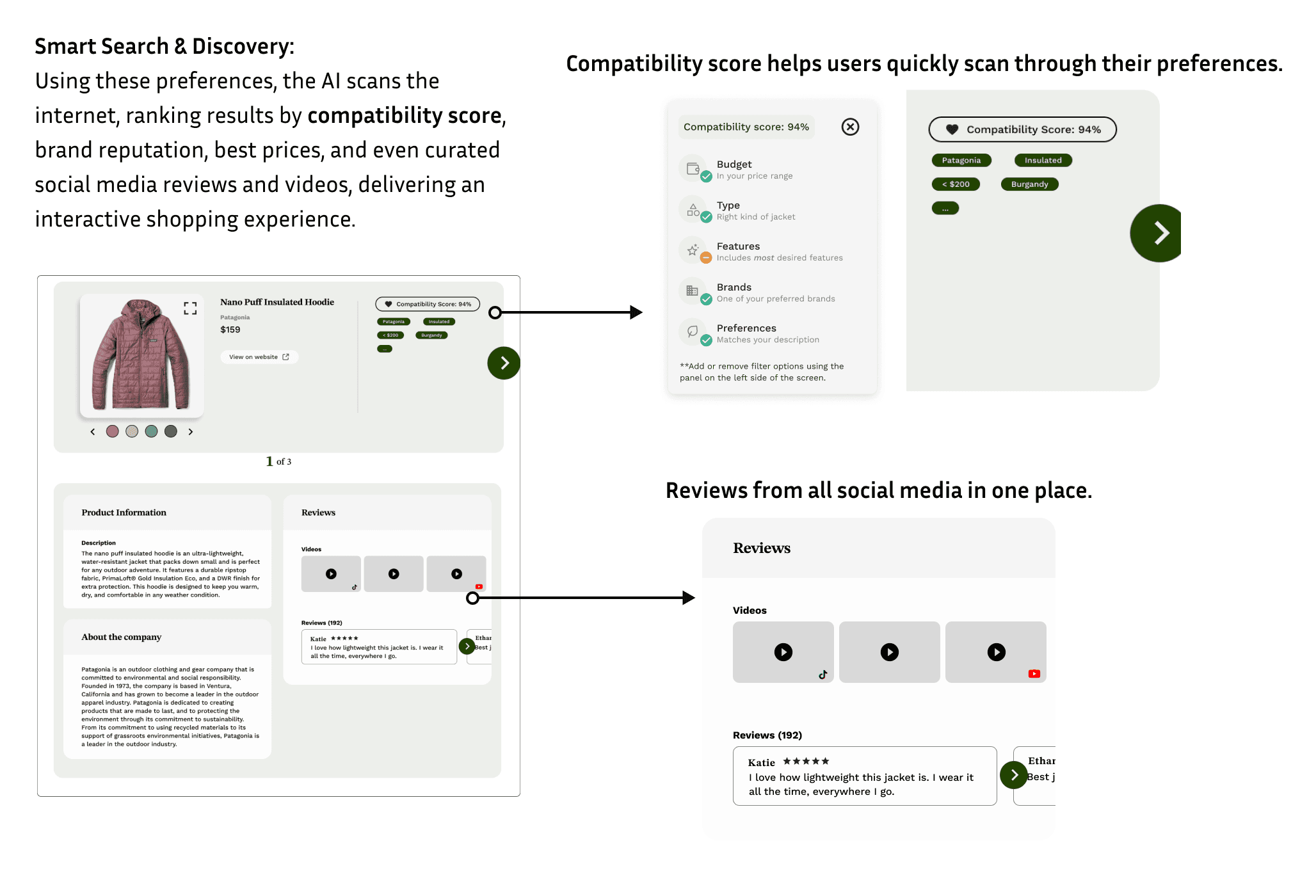
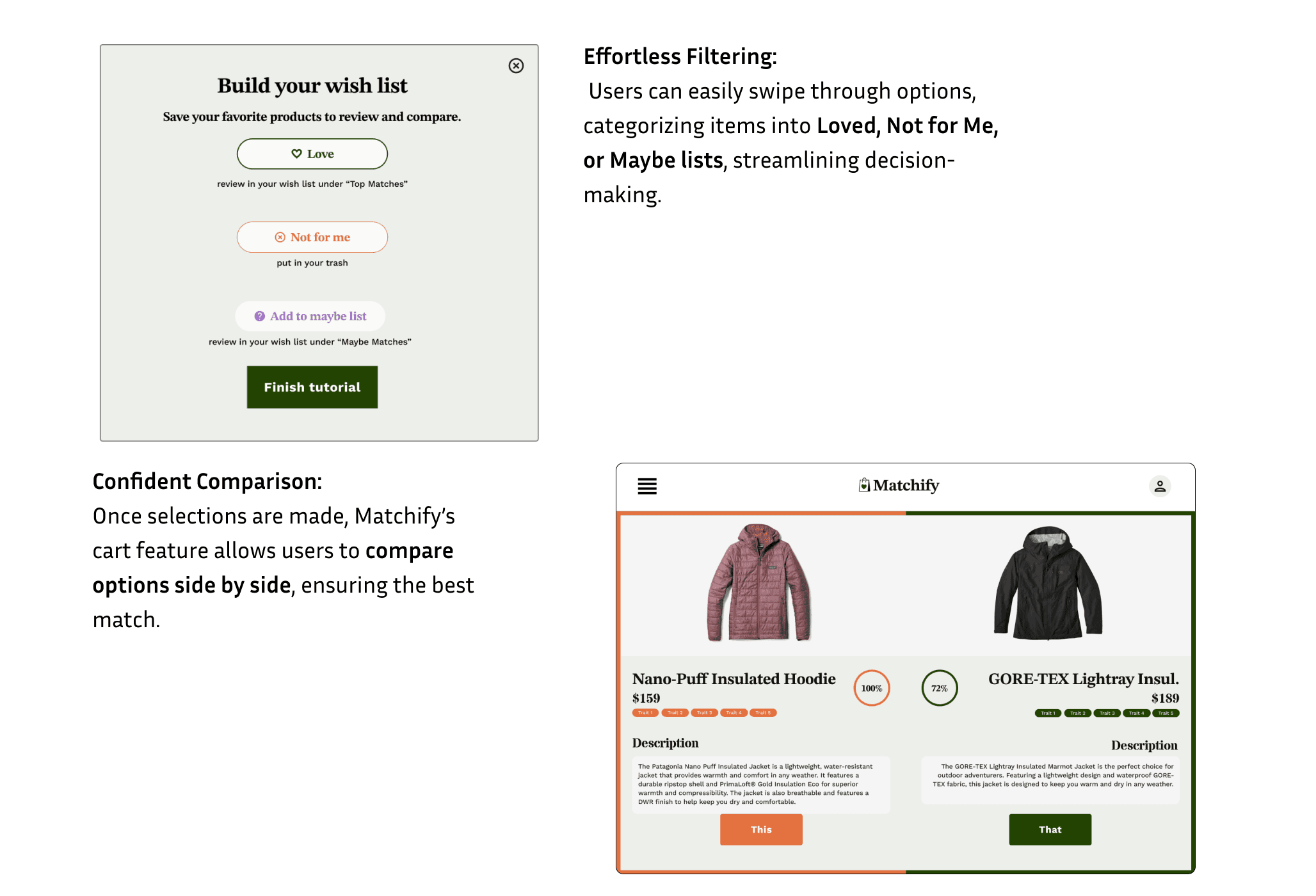
Lean UX Approach
Lean UX?
We have chosen a lean UX, an agile approach prioritizing speed, learning, and measurable outcomes over perfection. It focuses on creating a Minimum Viable Product (MVP) to enable rapid feedback and iteration.
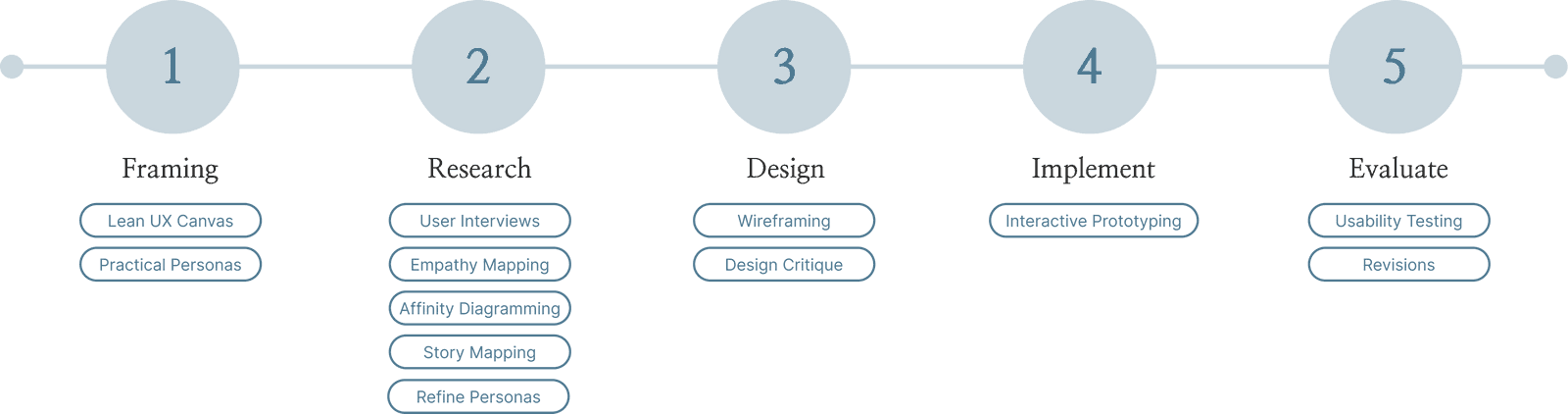
Lean UX Canvas
To first get an understanding of the underlying problem space surrounding online shopping, our team completed a Lean UX Canvas to identify the business problem, potential business outcomes, users, user benefits, and potential solution ideas. By working as a team to come up with different solution ideas, rather than hone in on one particular solution, we were able to explore many possible approaches to solving the problem at hand.
Practical Personas
Our practical personas were crafted prior to user interviews to help us keep our focus user-centric from early on in the process and explore what we thought we knew about users in the problem space. By crafting personas early on, we felt more confident about the types of participants we planned to recruit for user interviews, which would later be used to inform changes to our personas and refine our understanding. We crafted two persona profiles in order to foster a shared understanding of target users, including what we thought we knew and what we didn’t know. Below we share their background, goals, motivations, pain points, implications, and some context.
Imagine Sarah is looking to buy a new pair of running shoes. She starts by searching “best running shoes for beginners” on Google.
The search results show a mix of options:
Blog articles reviewing various brands.
Sponsored Ads from companies.
Videos on YouTube discussing features of popular running shoes.
Links to e-commerce platforms like Amazon, with reviews and price comparisons.
Sarah opens multiple tabs, each providing different details such as product features, user reviews, and pricing. However, she quickly feels overwhelmed by the sheer volume of information.
She finds it difficult to decide which source is reliable, what shoes meet her needs, and which option offers the best value. This choice overload and fragmented research slow her down, making her hesitant to proceed to the next stage of the shopping journey.
Research
While we believed consumers were experiencing difficulties while online shopping, we still wanted to validate if it would be worthwhile to explore the problem space in more depth. To do this, we dove into our initial primary research process, which utilized user interviews, empathy mapping, and story mapping, allowing us to collect rich, qualitative data and develop a deeper understanding about users’ experiences shopping online.
User Interviews
Research Method
To develop a deeper understanding of when and why consumers sometimes have a hard time deciding what to buy while online shopping, how they decide if they should buy an item, and when and why they return products, we conducted eight semi-structured individual interviews with users aged 22-46. We were intentional in recruiting at least 6 interview participants that aligned with each of our practical personas in order to validate and refine our understanding of target users.
Empathy Mapping
& Affinity Diagramming
Analysis Method
Using the data collected during user interviews, we categorized the information into empathy maps, which you can find a sample of below, to explore what users were saying, what their online shopping processes looked like, how they felt while shopping online, and what they think about the problem space.
Key Findings
Once our empathy maps were put together, the nuggets of information were pulled forward into an affinity diagram, where we could look for common themes and derive insights.
Challanges
Story Mapping
Synthesis method
Based on data collected during interviews, we also created a story map – a collaborative approach to condense into one artifact our understanding of the flow experienced by users as they move through their online shopping processes.
Working through our story map, we were also able to identify our MVP, helping our team align on the design aspects that would need to be prioritized and tested. Below is a list of the primary features that we planned to prioritize, as well as a sample of other important, but non-critical, feature ideas we could consider for a phase two iteration.
Design
Based on the problem space and our key research findings, our team came up with four primary design principles to help guide the design process.
Wireframing
With our research and design principles informing our design decisions, we were able to generate a series of wireframes as a starting point for the experience that would be offered by our solution. Each team member took time to develop solution mockups on their own, followed by a collaborative session where we came together as a team to discuss the options and choose the most promising and feasible option that would support our target users. Our early design approaches focused on implementing a personalized quiz feature to guide users through the process of sharing their preferences, offering a highly personalized experience.
Implement
Flow
Based on the feedback collected during the design critique session, our team got to work refining the design of our application and creating a prototype.
Evaluation
Usability Testing
With the first iteration of our prototype ready, we set out to collect another round of feedback, but this time from potential users. We conducted a series of six remote usability testing sessions with a diverse group of participants who aligned with Matchify’s target user groups and the practical personas we crafted.
Goals
From the usability tests, our team aimed to learn about three different research objectives:
Evaluation - Results
4 of the 6 participants were able to move through the task without assistance, but this left some room for improvement, which is where feedback from the participants came in handy.
As a result of the usability tests, we collected meaningful insights from participants, including:
Participants liked how they could have product information across different options all in one place through personalized recommendations and were excited by the possibility of doing their online shopping “under one roof”
The ability to compare products in-app and filter to refine options based on personal preferences was a valuable feature
There was some confusion about products that end up in the “wishlist” bucket and what happens to products that are saved for a later, possible purchase
When moving through the process of elimination, users wanted to be able to refer back to the compatibility score breakdown they saw while reviewing recommended options, as opposed to simply seeing the percentage